What Is An Epidemiological Model?
Today numbers and statistics dominate the media. Deceased, recovered, infected and other parameters inform us every day of the delicate situation of COVID-19 in the country. An epidemiological model helps us understand what the future holds, although it is not always right.
Those who showed interest in this issue before its globalization will recall that some studies reassured us with phrases such as “it is estimated that there will be no more than 10 infected in our country.”
When the virus was confined to Wuhan and its surroundings, the predictions were much more optimistic and no one was able to predict what would happen in the following months.
We have to be clear about one issue: it is not about media manipulation. Scientists and researchers rely on patterns in the here and now to predict, but minimal variables can drastically disrupt modeled results.
In this space we want to show you what an epidemiological model is and its variables, in order to understand the margin of human error and take the predictions discussed in the media with caution.
Modeling a catastrophe
The mathematical modeling of epidemics consists of the use of mathematics to explain and predict the behavior of infectious agents. They are usually deterministic models, that is, they assume that anyone can contract the disease at random.
Two main hypotheses can be established on which to build the models:
- The population of infected people is altered by their death or cure. Someone who is cured does not maintain the disease, so they are not cumulative values but variable over time.
- The rate of individuals who go from being susceptible to contracting the disease or being infected is proportional to the interaction between the number of individuals in both classes. Or what is the same: the more infected there are, the more susceptible the general population will be to contracting the disease.
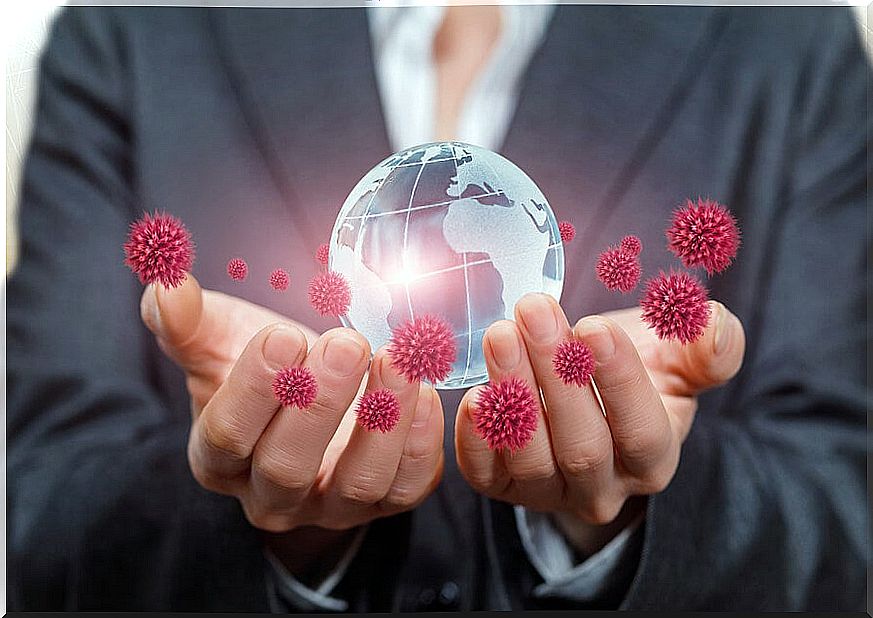
Numbers game against coronavirus
One of the simplest models to exemplify this theme is the SIR model. It is one of the most widely used epidemiological models due to its simplicity and compartmentalization of data. The parameters are simple:
- Susceptible population (S): people without immunity to the infectious agent who can become ill. Unfortunately, new diseases such as COVID-19 initially have a 100% susceptible population. The story is very different, for example with the flu, as the percentage of vaccinated people drastically decreases this value.
- Infected population (I): sick people who can potentially infect susceptible people.
- Recovered population (R): people who are immune to infection and consequently do not affect transmission when they come into contact with others. What is ironic is that many cases of the deceased are included in this parameter, since they cannot spread the disease.
The total population would be the sum of S, I and R. If we use these 3 compartments, through complex equations we can predict the fluctuation of people from one compartment to another over time. Sounds simple, right?… So why is it so difficult to make a reliable estimate?
The limitation of ignorance
Here we present a study by MedrXiv (Yale) conducted by a research team on January 28 about the spread of the virus. They themselves warn us of the limitations of mathematical modeling:
- The transmissibility of the virus is variable depending on the place and time it is used. The basic reproductive rhythm of the virus (R0) is between 2 and 3, and any slight variation in the parameter upsets the predictions.
- Many studies may cover only one medium of transmission. In the case of this study, only the transport of infected persons by flights is taken into account. But what about transport by car, on foot, by boat or by train?
- The effect of the measures taken by each country cannot be accurately predicted. Each nation acts differently against the virus. It is not possible to know when a country will decide to restrict movements, establish quarantines or close borders. You cannot model taking these measurements into account if you do not know when or how they will be taken.
In addition to all these complications, there is another that can be added:
- The recovered population (R), assumed in the SIR model as cured, may not be. Documented reinfection cases and asymptomatic carriers greatly complicate predictions. Therefore, early detection is essential.
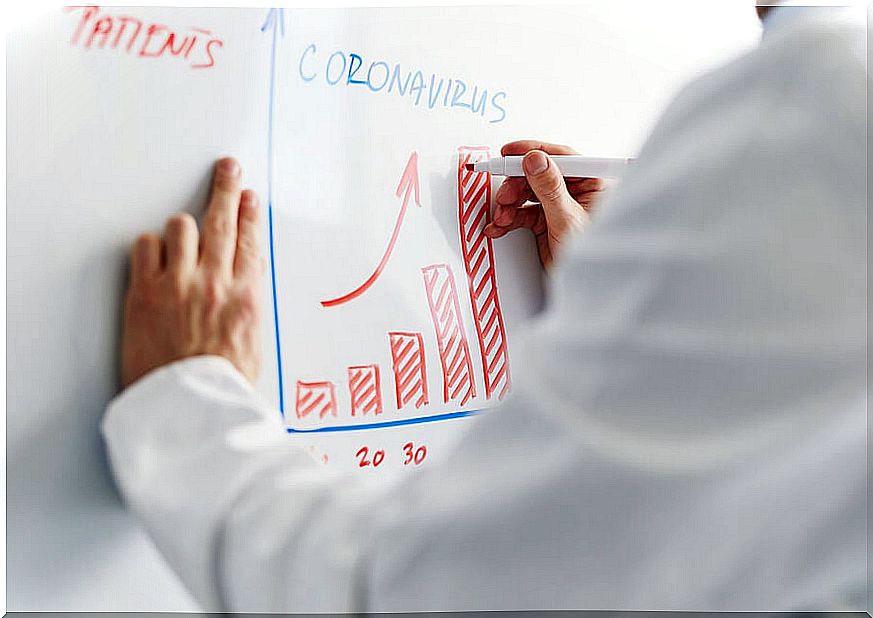
Positivity and caution
We hope that in this space the immense complexity involved in carrying out an effective epidemiological model has been demonstrated. The media and researchers try to provide the best possible information, but we must take the future figures they provide us for what they are: predictions.
For both good and bad, they can be wrong. But one thing is for sure, with the appropriate measures and the quarantine status, the spread of the virus will be slowed down sooner or later.